The Emergence of Contextual Finance
Listen to this article as a AI generated podcast. This podcast was created using NotebookLM from Google.
In recent years, the landscape of finance has undergone a significant transformation, moving away from traditional isolated functions towards a more integrated approach – contextual finance. I want to explore how these innovations like hyper-personalized financial activities and embedded finance,, are more efficient and responsive to the needs of individuals and businesses. By embedding financial services into everyday operations, individuals and organizations can navigate complex financial tasks with ease, ultimately enhancing their objectives.
In this article I will go through some concepts that are present today in different solutions as there are no intelligent omni-platforms present. I wanted to capture in one place, a vision of how future of finance can look like, given the tools and capabilities that are offered and developed today.
Finance has traditionally been treated as an isolated function. From applying for loans to making cross-border payments, organizations have had to navigate a patchwork of tools and portals, transferring data back and forth. But a new paradigm is emerging — Contextual Finance – CF (or contextual banking)—that promises to streamline these fragmented activities into an effortless, event-driven system.
In this model, individuals or companies no longer “perform” discrete financial tasks; instead, intelligent platforms weave financial services directly into day-to-day operations. A spike in product orders can trigger instant inventory financing; a low warehouse stock automatically requests replenishment from suppliers while factoring in exchange rates, shipping risks, and micro-insurance. These moments of financial need don’t have to be recognized and acted upon manually. The entire process, from detecting an operational cue to executing the necessary banking or payment functions, happens behind the scenes with minimal intervention.
By combining modular, API-based services from banks, fintech providers, payment gateways, and even NGOs, CF allows consumers and businesses to offload complexity—whether that’s cross-border settlements, credit underwriting, or charitable donations.
Advanced analytics and event-driven architecture provide real-time oversight, ensuring compliance checks are run automatically, fraud risks are mitigated, and decisions are auditable for regulators. The result is a frictionless environment where finance supports strategic objectives rather than creating a to-do list of separate tasks.
This shift is not simply about plugging in new software. It’s about rethinking the role of finance itself—no longer a separate domain but a seamless, context-aware layer that sparks to life whenever the business needs it. Over the following discussion, we’ll explore how this vision of FaaS and contextual finance is reshaping the way companies handle money, from supply chain logistics to front-end customer experiences.
Growing enterprises often find their ambitions hamstrung by the complexity of managing money. Departments navigate a maze of disparate systems for payments, lending, insurance, and compliance, leaving staff to piece together each financial step manually. Everything from cross-border transactions to routine invoice approvals can turn into a bottleneck. In this evolving landscape, organizations are beginning to envision a future where finance is no longer a separate chore, but a seamlessly integrated part of everyday operations—proactively meeting each business need the moment it arises.
The Essence of Contextual Finance
At its core, contextual finance is about embedding money management right into our everyday activities, whether personal or business-related. Traditionally, you’d head to a bank (physical or digital) whenever you needed to complete a specific transaction—transfer funds, open an account, apply for a loan. In a contextual finance world, the bank effectively comes to you – or it comes at the place where transaction is happening – hence the “finance” part.
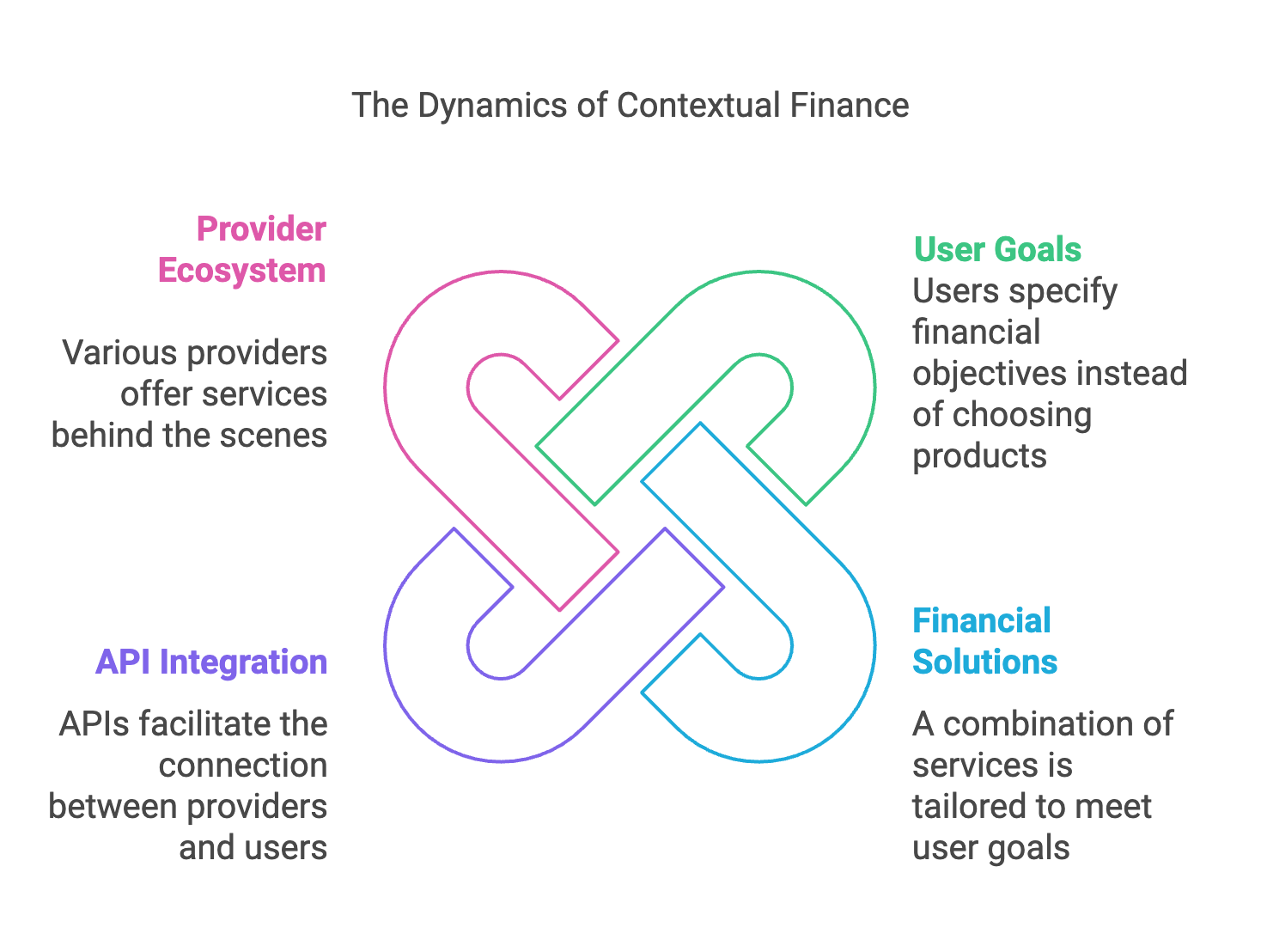
Goals Over Products
Instead of scanning a menu of products—checking accounts, credit cards, term loans — users specify their goal: “I need to restock inventory,” “I want to donate monthly to a climate initiative,” or “I’m financing a family vacation.”
The platform automatically pulls the right combination of financial solutions from its partner ecosystem.
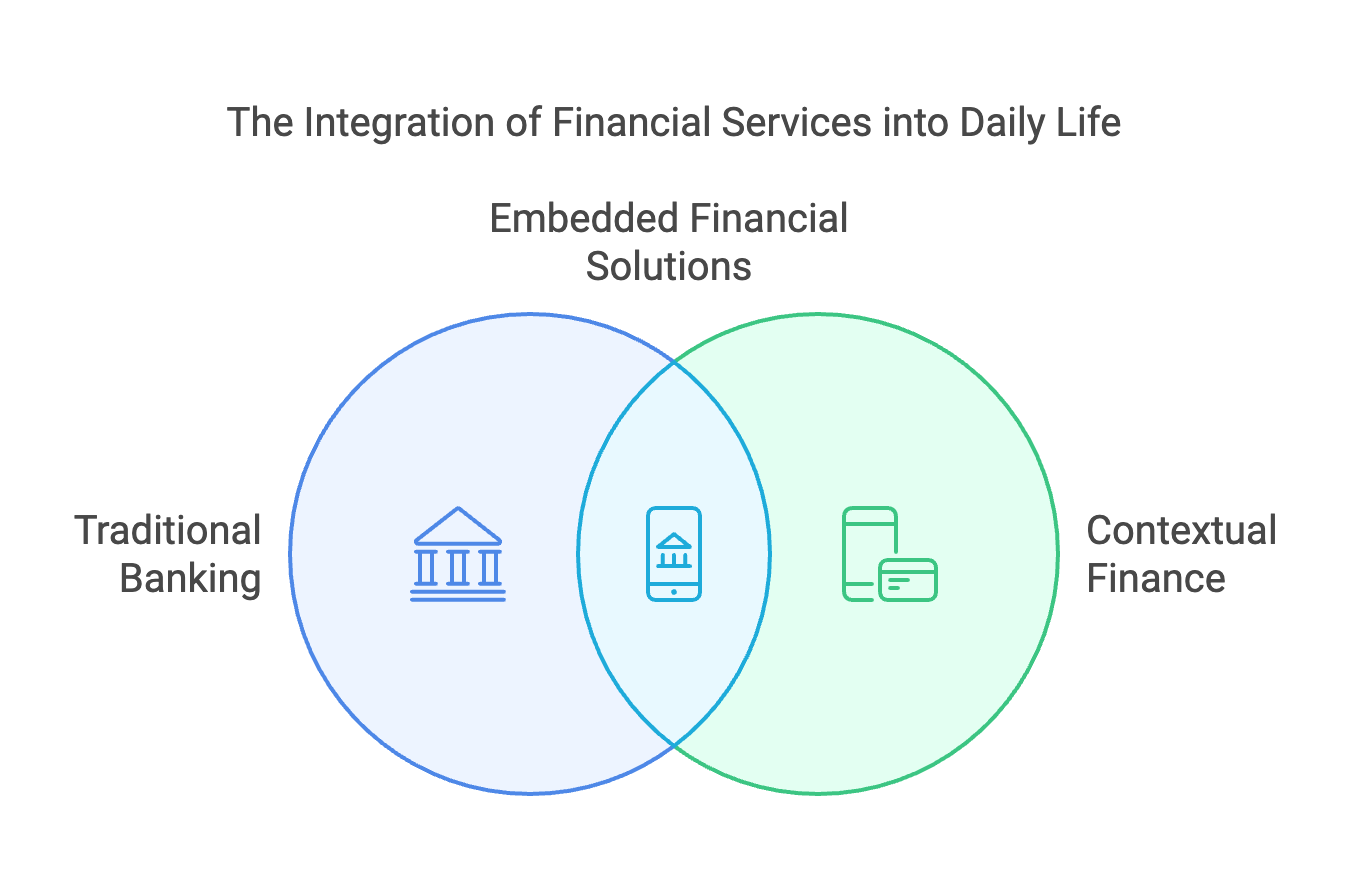
Embeded Finance
Integration opens up these services—payments, lending, insurance, charitable giving—via APIs. Multiple providers (banks, NGOs, micro-lenders) plug in behind the scenes.
When triggered by user behavior (like low inventory or a travel booking), the platform arranges the best option in real time.
Rise of AI & ML
As automation becomes more deeply woven into how organizations and consumers manage their finances—orchestrating tasks from credit decisions to intelligent cash flow tracking—artificial intelligence (AI) and machine learning (ML) have emerged as the game-changers behind the scenes. These technologies add a layer of predictive power and adaptability that goes far beyond simple rule-based automations.
AI-driven models can, for instance, interpret massive data streams—from operational metrics like inventory turnover to user behavioral patterns in online commerce. By crunching real-time statistics, ML algorithms can spot shifts in demand before they fully materialize, prompting an automatic reallocation of funds or pre-approval of a short-term loan. They also excel at nuanced risk profiling: instead of relying on one-size-fits-all credit checks, ML can consider a broad set of indicators—sales frequency, supplier reliability, seasonality—to deliver instant and more personalized financing options.
Beyond lending, AI and ML enhance fraud detection and compliance, swiftly flagging suspicious patterns that might have gone unnoticed in a manual review. As companies extend their reach across multiple regions and payment rails, these safeguards adapt to each market’s unique requirements and norms. Ultimately, this intelligence layer allows everything from e-commerce storefronts to industrial logistics platforms to function more like living ecosystems—anticipating, reacting, and adapting in real time to minimize friction and maximize opportunities.
Agentic Finance
The concept of “Agentic Finance” builds upon embedded, context-driven, and AI-enabled solutions to create an ecosystem where financial services actively work on the user’s behalf. Rather than existing as passive tools that require manual input or routine maintenance, these platforms behave more like specialized agents—capable of monitoring real-time data, interpreting user-defined preferences, and executing actions automatically when certain conditions arise.
In practice, agentic finance might detect that a growing business’s cash flow is under pressure due to a sudden spike in orders. Without waiting for manual intervention, the system could secure a short-term credit line from the most suitable provider—factoring in interest rates, approval speed, and alignment with the company’s sustainability guidelines. The platform simultaneously checks inventory levels, triggers a restock request to the chosen supplier, and ensures any currency exchange is performed at an optimal moment. All these steps happen under user-defined constraints, giving the system clear marching orders while still leaving final oversight to humans when needed.
Much of the intelligence behind this is powered by advances in AI and ML. The platform continuously analyzes patterns—like revenue dips during off-seasons or user preferences for local manufacturing partners—and develops agentic behaviors. If a retailer prioritizes eco-friendly suppliers, the agent will not only filter out partnerships that fail to meet environmental criteria but also proactively suggest financing structures that reward sustainability efforts. This goes beyond workflow automation; it’s a fluid system that “thinks” about what’s best for the user’s objectives.
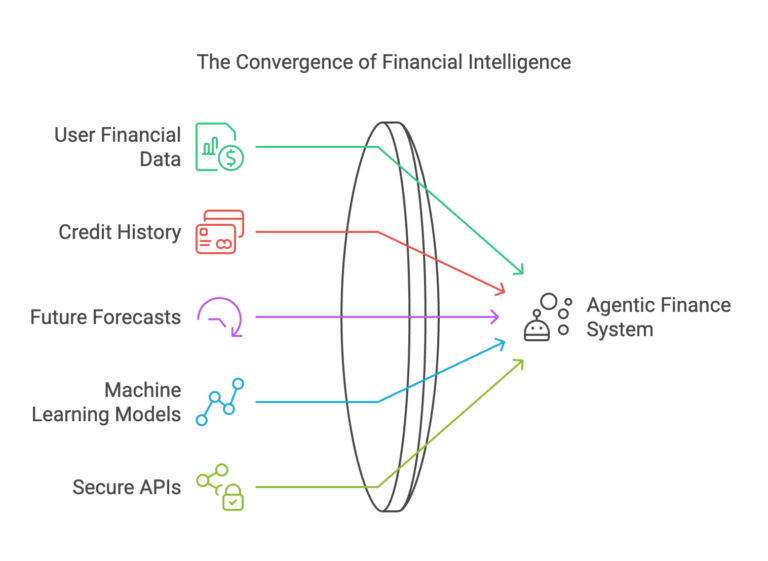
Agentic finance removes layers of friction by minimizing guesswork. Instead of the user having to request a loan, fill out forms, or compare rates across multiple sites, the agent already has a holistic view of the user’s financial data, credit history, and future forecasts. When the system detects a viable opportunity or challenge—whether it’s a great spot to secure funding or an early warning for a potential shortfall—it steps in as an active participant. Humans remain in the loop for high-level direction and final approvals, but the routine legwork of scanning, evaluating, and initiating has migrated to a self-acting layer.
For end users, “Agentic Finance” can feel near-magical: like having a financial concierge on standby 24/7. But beneath that magical exterior lies a rigorously architected framework of machine learning models, secure APIs, and continuous event processing. Banks, fintechs, and data providers all plug into a central engine, which acts as an orchestrator among them. Each agentic decision leaves behind an auditable trail, ensuring transparency for regulators and peace of mind for users who want to understand why the system took a particular action.
By design, agentic finance transcends the boundaries of traditional banking products to include everything from automated insurance coverage triggers to carbon-offset micro-donations. The key is that this “agency” always remains in service to the user’s goals—like stable cash flow, sustainable sourcing, or cost minimization—and carries out routine financial decisions that would otherwise be time-consuming or cumbersome to manage. As we continue to embrace AI-driven platforms, this agentic layer is poised to become the backbone of how businesses and individuals handle the complexity of money in a deeply interconnected world.
Hyper-personalized automated finance management
The logical extension of agentic, context-driven solutions is the rise of hyper-personalization in automated finance. No two individuals or businesses have the same needs, risk appetites, or financial goals—and hyper-personalized finance management ensures that every automated action, from underwriting to subscription renewals, aligns with each user’s unique situation. Rather than generic “one-size-fits-all” settings, advanced algorithms and data analytics craft bespoke financial pathways for every participant.
In practice, hyper-personalization might take the form of dynamic interest rates based on a user’s real-time performance indicators or external market shifts. A small enterprise experiencing a seasonal lull could automatically receive a lower repayment schedule for a micro-loan, reducing the chance of default and fostering long-term loyalty. Once revenues pick up, repayments gradually scale, all without forcing the business owner to renegotiate new terms. These individualized adjustments can also include aligning portfolio strategies for personal investments—purchasing green bonds if a user prioritizes sustainability or funneling spare change into micro-investments for those focused on long-term growth.
Under the hood, the data powering hyper-personalized automation often includes everything from transaction histories and supply chain analytics to lifestyle preferences and social cues. Machine learning models ingest this information, formulating patterns and predicting future actions. One user might opt for risk-averse saving strategies if they’re nearing retirement or preparing for a property purchase, while another might channel available funds into a “high-risk, high-reward” portfolio aligned with entrepreneurial ambitions. The platform ensures these choices manifest instantly in every financial interaction, whether it’s paying supplier invoices or allocating leftover cash from monthly revenues.
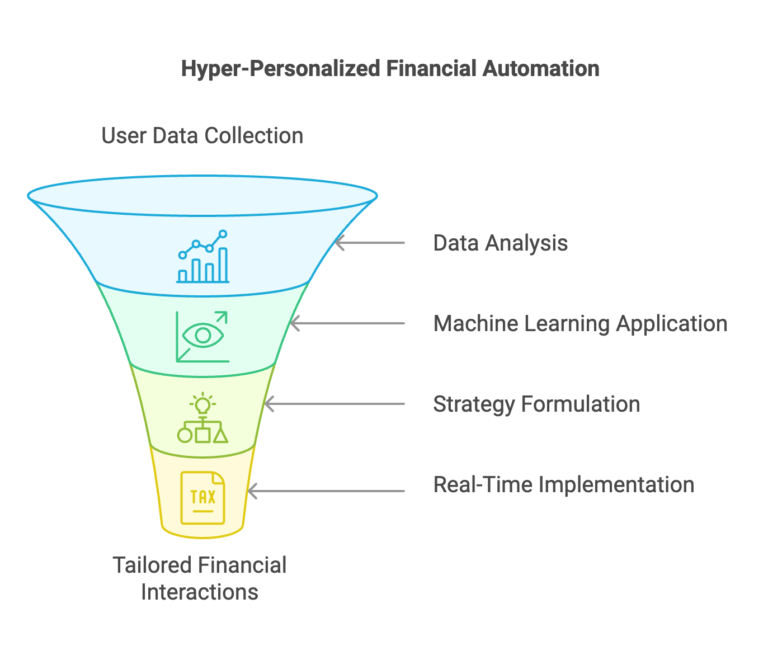
For end-users, hyper-personalization eliminates the drudgery of constantly reconfiguring or evaluating their financial plans. Once they set overarching preferences—like sustainability goals, growth targets, or risk limits—the system applies those preferences in real time. Meanwhile, continuous data processing checks for opportunities to optimize, like better interest rates, relevant insurance products, or timely investment switches that can elevate returns or reduce overhead. This tailored approach not only saves time but also fosters deeper engagement and trust, as users see tangible results that reflect their priorities rather than generic market offerings.
Context-aware finance and hyper-personalized automated finance management encapsulates the promise of next-generation financial ecosystems: a future where economic decisions adapt at the speed of life, effortlessly blending user insights with powerful, context-aware technology. By handling the nitty-gritty through dynamic, data-driven personalization, these platforms let people and businesses concentrate on their bigger goals—whether that’s expanding into new markets, improving sustainability footprints, or simply enjoying greater peace of mind.
Let's connect
Context-Driven Financial Architecture (CDFA)
Beneath the surface of automated lending decisions, proactive cash flow adjustments, and on-the-fly currency conversions lies an architectural blueprint designed to handle massive volumes of data, events, and complex orchestration. This framework is what we call Context-Driven Financial Architecture (CDFA)—a technology paradigm built to ensure that every financial action, from micro-payments to long-term credit deals, is shaped by real-time context rather than rote processes.
CDFA starts with an event-driven core, where triggers like “inventory low” or “funds insufficient” set off a series of interlinked processes involving analytics engines, microservices, and integrated third-party APIs. It interweaves various data streams—sales, operational metrics, user behaviors—and instantly filters them through AI and ML models. By doing so, it avoids one-size-fits-all rules and instead delivers nuanced, situational responses. If a retail platform flags a surge in demand, CDFA not only handles dynamic pricing or promotional strategies but also aligns financing (like short-term inventory loans), insurance coverage for shipping risks, and currency hedges if orders come from overseas.
Crucially, every service or microservice in a CDFA is modular. Lending, payments, fraud checks, and ESG monitoring each run in their own domain, yet they communicate via secure, event-based protocols. This makes it possible to scale specific functionalities or add new partners—say, a green financing provider—without rewriting entire systems. Compliance becomes a matter of real-time checks with an auditable event log, sparing teams the headache of manual oversight.
Above all, CDFA ensures the user or business doesn’t merely benefit from one-off automations; it creates a holistic, situationally aware ecosystem. Whether it’s a SME anticipating seasonal stock needs or a global enterprise mitigating currency risk, the architecture layers each financial interaction with timely, context-aligned intelligence. The result is a living system—always listening, constantly learning, and perpetually adapting to maximize both efficiency and user satisfaction.
Contextual Finance Engine (CFE)
Where Context-Driven Financial Architecture (CDFA) represents the broader blueprint for orchestrating financial processes in real time, the Contextual Finance Engine (CFE) is the operational heart that powers those orchestrations. Acting much like the central nervous system in a living organism, the CFE sits at the nexus of data, triggers, and user directives—coordinating an array of microservices and third-party integrations to produce timely, context-aware financial actions.
A well-designed CFE continually ingests signals from disparate sources, such as sales platforms, IoT sensors, inventory systems, or user activity logs. It leverages AI and ML models to interpret these signals: identifying a potential need for short-term financing, automating a currency conversion at an optimal rate, or suggesting an ESG-friendly investment option. Crucially, these calculations and decisions are neither static nor dependent on a singular data feed. The engine’s adaptability means that as new datasets or partner services come online, the CFE can integrate them seamlessly, enriching its decision-making capacity.
Perhaps the most compelling facet of a CFE is its ability to execute complex, multi-step workflows with minimal human intervention. For example, if a shipment is delayed in transit, the engine can automatically extend a micro-loan to cover cash flow gaps, ping insurance services to update coverage, and recommend reordering supplies before stockouts occur. Everything happens according to pre-defined rules and machine-learning insights, yet remains flexible enough to accommodate unforeseen variables or updated user preferences.
Ultimately, the CFE brings the promise of contextual finance to life. It ensures that no transaction, alert, or service request exists in isolation; instead, each is processed through a lens of real-time context, delivering a level of personalization and orchestration that static or siloed financial systems simply can’t match. By uniting data, automation, and analytics under a single event-driven framework, the Contextual Finance Engine represents a major leap forward in how both businesses and consumers interact with finance on a daily basis.
How CFE Fits into CDFA
Within the Context-Driven Financial Architecture (CDFA), the Contextual Finance Engine (CFE) acts as the real-time operational core that brings context-aware decisions to life. CDFA itself is the high-level architectural framework—encompassing event-driven communication, microservices design, compliance mechanisms, and data governance rules. It sets the stage for how different components, services, and partners interact, ensuring the entire ecosystem can handle dynamic financial workflows at scale.
Meanwhile, the CFE is the system’s “intelligent conductor.” It continuously ingests data from across the CDFA—such as inventory levels, currency fluctuations, user behavior, or market trends—and applies AI and ML models to interpret these signals. Based on that interpretation, the CFE automatically orchestrates the relevant microservices and workflows, whether it’s initiating a short-term loan, pre-emptively adjusting currency hedges, or recommending ESG-friendly financing. Essentially, CDFA provides the “blueprint and infrastructure,” while CFE supplies the “event-driven intelligence” that transforms raw data and user-defined rules into context-aware actions.
The synergy between the two is what drives next-level automation and personalization. CDFA ensures all the architectural pieces fit together securely and efficiently, and the CFE leverages that foundation to execute seamless, real-time decisions. By dividing the responsibilities—architecture at the CDFA level and contextual orchestration at the CFE level—organizations can rapidly adapt to changing conditions, integrate new financial partners or data sources, and scale on demand, all while maintaining a single source of truth for every event and transaction.
Graphics made using napkin.ai