Are we content being users of someone else’s AI, or do we want to be owners of our AI destiny?
AI thinker Tweet
Generative AI has stormed into the enterprise mainstream. In the wake of breakthroughs like GPT-4, organizations are racing to infuse AI into products and processes. Yet amid this excitement, IT leaders face a strategic dilemma: should you rely on third-party AI services, or build and own your own AI platform? For many forward-thinking enterprises, owning your AI platform is emerging as a key competitive advantage. By retaining control of both public large language models (LLMs) and proprietary models in-house, organizations can innovate faster without compromising on security, compliance, or flexibility. This approach enables companies to harness powerful public AI services when needed and develop domain-specific AI models – all while keeping sensitive data under their own roof.
Long text ahead !
TL DR :
Owning your AI platform, such as Architech’s Archy, strategically positions enterprises to securely leverage both public and proprietary generative AI models without risking data breaches or vendor lock-in. Archy’s open, integration-friendly architecture allows businesses to seamlessly incorporate AI into existing workflows, significantly accelerating the transition from business requirements to actionable product backlogs—boosting productivity and shortening time-to-market. Unlike closed or SaaS-only AI solutions, Archy gives enterprises full data control, customization capabilities, predictable costs, and flexibility to adopt and evolve AI capabilities at their own pace, ensuring secure, compliant, and future-proof generative AI adoption tailored specifically to enterprise needs.
Owning an AI platform means treating AI infrastructure as a core enterprise asset, much like your cloud or data platforms. Rather than sending proprietary data off to a vendor’s black-box API, you bring the AI to your data on your terms . The result is a secure, open foundation that lets you adopt generative AI at your own pace and integrate it deeply into your business. This article explores why owning your AI platform delivers strategic benefits, how Architech’s open architecture exemplifies this approach, and how it accelerates product development from requirements to reality.
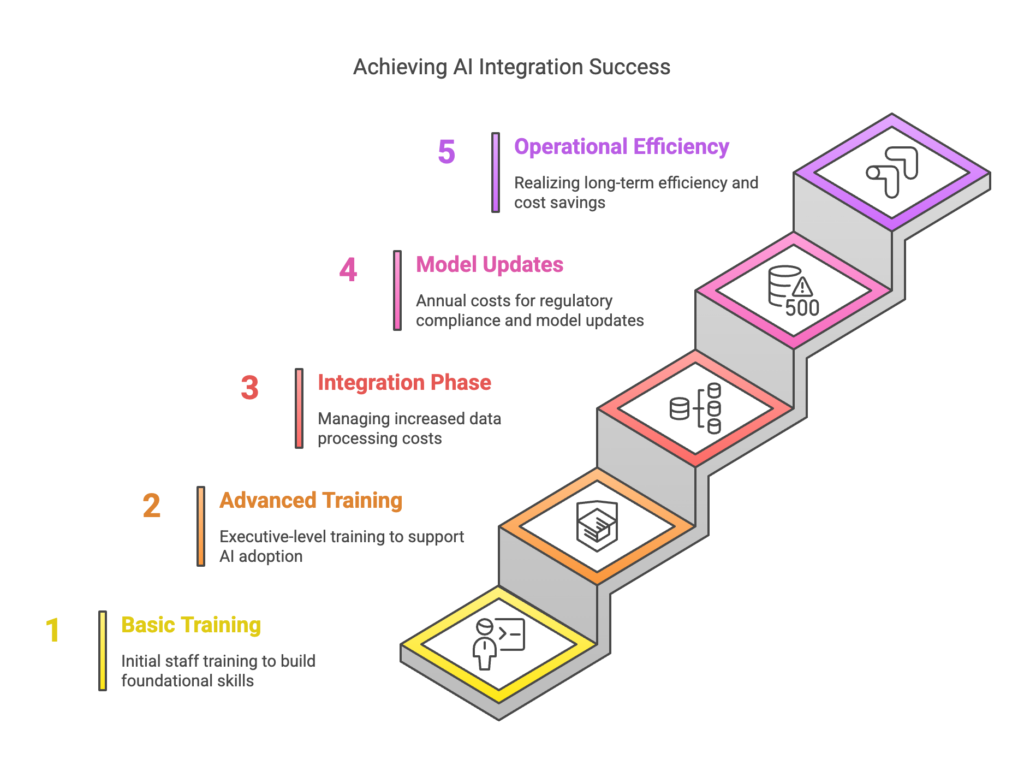
The Strategic Case for Owning Your AI Platform
Building an AI platform in-house or adopting an open platform like Archy (Architech’s enterprise AI assistant) requires upfront investment and vision. But the payoffs are substantial in several key areas:
Data Sovereignty and Security
Handing your data to a third-party AI service means entrusting them with your crown jewels – something many CIOs are rightly wary of. A subscription-based AI model leaves your data security in the hands of an external provider that may not prioritize it . In contrast, owning your platform grants full sovereignty over your data, with complete control over where data is stored and how it’s processed . You no longer have to simply trust a vendor to keep sensitive information safe – you maintain end-to-end oversight within your firewall. This ensures strict compliance with regulations like GDPR and HIPAA, since you can enforce all required safeguards internally. In short, AI ownership means no data leaves your trusted environment, eliminating the risk of leaks or unauthorized access .
Flexibility and LLM Choice
Another major advantage of owning the platform is freedom from vendor lock-in. Many AI SaaS offerings tie you to one model or cloud service – for example, a proprietary chatbot that only uses its creator’s LLM. This one-size-fits-all approach often fails to align with an organization’s specific needs . By contrast, when you control your AI environment, you have your choice of models and can even deploy multiple LLMs fit for different purposes . You’re free to experiment with the latest OpenAI GPT series, Google’s models, open-source LLMs like LLaMA, or a bespoke model fine-tuned on your proprietary data – whatever best suits the task . Vendor-provided solutions may lock you into a specific model, but an owned platform lets you mix and match or swap models as you see fit . Crucially, those models can also be fine-tuned to your industry’s terminology and datasets for superior results . This flexibility means you can adapt to new AI advancements on your timeline, rather than waiting on a provider’s roadmap or being stuck with yesterday’s tech .
Customized Capabilities and Integration
Off-the-shelf AI tools are generic by nature. They might perform adequately out of the box, but they aren’t tailored to your business processes. Owning the platform allows you to purpose-fit AI solutions to your objectives, increasing productivity across departments . You can develop custom AI workflows (for example, automating financial report generation or triaging support tickets) that align with your internal workflows and data. This ability to embed AI deeply into your operations is a huge efficiency gain – AI isn’t a separate tool, it becomes an integral part of your enterprise architecture. In addition, an open platform encourages integration with your existing systems. Rather than a siloed application, the AI services can connect to your data lakes, knowledge bases, and business applications via APIs and event streams. As we’ll see with Archy, an open architecture makes it much easier to weave AI into the fabric of your IT landscape, so teams can leverage AI within the tools they already use.
Predictable Cost and Control
From a financial perspective, owning your AI stack can also offer more predictable costs and better ROI in the long run. Pay-as-you-go cloud AI APIs often result in escalating, unpredictable expenses – costs per token or per call can skyrocket as usage grows . Enterprises have faced surprise bills when AI adoption expands faster than expected. By contrast, investing in an in-house or private platform means upfront known costs (infrastructure, licenses, maintenance) but fewer “metered” surprises. It’s easier to budget when you’re not subject to an external provider’s pricing changes or hidden fees . Additionally, you gain full control over scaling – you can optimize hardware and deployments for your workload without vendor-imposed limits or costs. For executive stakeholders, this cost predictability and transparency are important strategic advantages that free up budget for other initiatives.
Owning your AI platform gives you greater control, security, customization, and flexibility than outsourcing this core capability. As one industry analysis put it: “By owning your AI platform, you can have full sovereignty over your data” and shape the platform to meet your needs . It’s a proactive strategy to make AI a long-term, well-governed asset for the enterprise, rather than a quick fix you have limited say in.
Leveraging Public and Proprietary LLMs – Securely
One of the powerful aspects of a platform like Archy is the ability to get the best of both worlds: leverage public LLMs where they make sense, and develop proprietary AI models for your unique needs – all under a secure umbrella. Many organizations want to experiment with state-of-the-art public models (such as GPT-4 or Claude) given their impressive capabilities. At the same time, they have proprietary data and domain knowledge that could benefit from custom-trained models. Owning your platform lets you do both, without exposing your data to outside parties.
How is this possible? The key is architectural: instead of sending your data out to an external AI service, you bring the AI to your data. That might mean deploying open-source LLMs on your own cloud infrastructure, or using private endpoints for a hosted model so that the provider never sees your raw data . In Archy’s case, the platform can be deployed within your Azure cloud tenant, and it leverages Azure’s AI services (like Azure ML Studio or AI models you configure) in a way that keeps all data processing internal to your environment . Your prompts, documents, and knowledge bases remain behind your firewall; only the model’s outputs are returned to your applications. This approach mitigates data privacy and residency concerns. As one expert noted, “Data sent externally can violate policies like GDPR”, so avoiding sending sensitive data to external LLM APIs is critical . By containing AI workflows inside your secured cloud or on-premise setup, you eliminate that class of risk.
Security professionals also worry about the opaque nature of public AI services. There’s little transparency into how third-party LLM providers handle or store the data you send them – these algorithms are essentially black boxes . Furthermore, you have no control over where your data might travel or be replicated in the process of using a public API; in the worst case, “data could land on insecure servers” outside your oversight . An owned platform like Archy sidesteps these unknowns. You define the security measures (encryption, access control, monitoring) and retain complete visibility into data flows and model behavior. Archy, for instance, supports deploying in air-gapped or isolated environments and inherits your existing security policies – meaning it conforms to your enterprise’s security posture rather than introducing new vulnerabilities.
Critically, owning the platform doesn’t mean foregoing the benefits of public AI innovation. You can still tap into cutting-edge models – but you do so in a controlled, hybrid manner. For less sensitive tasks or publicly available data, you might call an external API via a secure gateway. For anything involving regulated or proprietary data, you use your in-house model or a fine-tuned version of an open model running locally. The platform orchestrates this seamlessly. Over time, you might even reduce reliance on third-party models as your proprietary LLMs become more capable, trained on your data. Many enterprises pursue this hybrid strategy initially: use a mix of vendor models and internal models, then gradually specialize. With Archy’s open architecture, such a hybrid approach is supported by design – you are free to plug in new models or switch providers as the landscape evolves. The result is AI agility with security, allowing you to leverage the best models available while rigorously protecting your data assets.
Open Architecture and Integration at Your Own Pace
Technology leaders know that big-bang platform replacements rarely succeed. One of the strengths of owning your AI platform is the ability to adopt and evolve AI capabilities at the pace that suits your organization. Architech’s design philosophy embraces open architecture and deep integration, ensuring that introducing AI augments your existing ecosystem rather than disrupts it. In practical terms, an open architecture means the AI platform can plug into your current tools, data sources, and workflows with minimal friction – so you can start with incremental use cases and gradually expand.
Archy demonstrates this with its integration capabilities. It is not a standalone app that forces you to migrate your data or abandon established tools. Instead, Archy acts as an AI layer that sits on top of and alongside your project management and product development tools. For example, Archy integrates natively with Atlassian Jira as a plugin . Product managers and teams can continue using Jira for tracking backlogs and issues, while Archy works within Jira to provide AI-driven assistance. This means zero context-switching – the AI comes to your workflow. Similarly, Archy connects with Confluent for real-time data streaming , allowing it to incorporate live data and events into its insights. It also supports custom API integrations , meaning if you have other internal systems (say a requirements database or a knowledge wiki), Archy can hook into those to gather input or push updates. The composable, open architecture makes it possible to integrate Archy with your existing infrastructure “at your own pace, ensuring smooth adoption.”
This flexible integration is key for enterprise readiness. You might choose to start by deploying Archy for a single team or project as a pilot – plugging it into that team’s Jira project and data sources. Because Archy runs in your environment (for instance, in your Azure cloud), you can limit the scope and safely trial its capabilities. Once it proves value, you can scale up to more teams or use cases, gradually weaving AI assistance into more product workflows. The open architecture supports this incremental rollout; you’re not forced into an all-or-nothing migration. Additionally, because Archy leverages your existing cloud services (like Azure AI and your security framework) , your IT team can manage it with familiar tools and governance processes. This greatly eases adoption – compliance officers, architects, and ops teams are on board because the solution extends what you already have, rather than introducing a completely foreign system.
Contrast this with many “closed” AI platforms where you must use their interface or cloud service in isolation. Those might require you to export or duplicate data into their system, or only work with a narrow set of tools. Such approaches can be rigid and force organizations onto the vendor’s terms and timeline. Architech purposely took a different route: by being integration-friendly, it allows enterprises to embrace AI on their own terms. Whether you want to start small or go enterprise-wide, the platform adapts. As one AI architecture principle states, “Composable, open architecture [lets you] integrate an AI fabric with your existing infrastructure at your own pace” – this ensures your AI adoption is smooth and aligned with your readiness. The bottom line is that an open AI platform becomes a natural extension of your enterprise architecture, not a disruptor. This positions you to continuously evolve your AI capabilities, swapping in new models or connecting new data sources as needed, without re-architecting from scratch.
Accelerating Product Development from Requirements to Reality
Perhaps the most exciting advantage of owning your AI platform is how it can directly accelerate the delivery of customer value. One high-impact use case across industries is using AI to supercharge the product development lifecycle – especially the early stages of requirements gathering, analysis, and backlog creation. Architech’s Archy assistant is purpose-built for this scenario: it dramatically shortens the journey from business idea to actionable product backlog. This capability is a game-changer for product managers and IT leaders striving to deliver features faster and more efficiently.
Consider the traditional process of going from vague business requirements to a concrete list of user stories and tasks. It often involves multiple lengthy workshops, countless email threads to clarify needs, and manual drafting of specification documents or Jira tickets. Weeks can be spent translating stakeholder wishes into well-defined epics and user stories for the development team. Archy compresses this timeline by using AI to automate much of that translation work. It can ingest high-level inputs – project charters, requirement documents, even conversation notes – and generate initial epics, user stories, and acceptance criteria that align with the business needs . In essence, Archy serves as an AI co-pilot to the product manager, turning natural language descriptions of goals into structured backlog items.
For example, a product manager could input a plain-language description like “We need a mobile app feature that allows customers to deposit checks by taking a photo, with real-time fraud detection”. Archy would analyze this and produce a set of epics and user stories such as “Mobile Check Deposit Feature” with user stories for capturing check images, verifying check details, integrating a fraud detection service, etc., each with draft acceptance criteria. It might suggest “As a banking customer, I want to deposit a check via the app so that I don’t have to visit a branch” as a user story, with acceptance criteria like “Given a clear check photo, the system recognizes the amount and payer correctly”. These are starting points that the product team can then review and refine. The AI essentially kickstarts the backlog creation, doing in minutes what might have taken days of meetings and documentation.
This acceleration has a direct impact on time-to-market. By quickly generating a detailed and comprehensive backlog aligned with the project goals, Archy allows development to begin earlier . Teams can focus their time on refining and executing the work, rather than on tedious initial writing. One source notes the time efficiency of AI-generated user stories – it “quickly generate[s] detailed user stories, allowing teams to focus on … backlog refinement” rather than starting from scratch. In practice, this means your developers and testers get clarity sooner, and stakeholders see working software faster.
Another benefit is improved quality and clarity of requirements. Archy’s AI doesn’t just speed up writing; it also brings consistency and thoroughness. It suggests standard formats (e.g., the classic As a [user]… I want… so that… form for user stories) and can ensure that acceptance criteria are present for each story. By analyzing lots of data, it can remind teams of edge cases or non-functional requirements they might overlook. For instance, Archy can propose acceptance criteria covering performance (“the system should process a check in under 5 seconds”) or edge cases (“the photo is blurry or the check is already deposited”) that make the backlog more robust. It’s like having an encyclopedic analyst on the team who has seen thousands of projects – the AI draws on that generalized experience to strengthen your requirements. The result is fewer gaps and ambiguities, which in turn leads to fewer misunderstandings down the line. (In fact, Archy’s backlog assistance capability learns from user feedback over time, getting even more attuned to a team’s domain and preferences with each use .)
It’s important to note that this AI-driven backlog creation is applicable across industries. Whether you’re in finance, healthcare, retail, or tech, the challenge of turning business needs into implementable user stories is universal. Archy’s model can be fine-tuned with industry-specific knowledge – for example, using a bank’s past project data to become fluent in banking terminology, or training on healthcare use cases to understand medical compliance needs. Owning the platform makes such fine-tuning feasible, since you can train the AI on your domain data securely. As mentioned earlier, having your own AI allows models to be customized to your terminology and workflows . This means the AI’s output becomes even more relevant and accurate for your field. A telco company could have Archy imbibe telecom-specific user story patterns; a manufacturing firm could feed Archy its repository of requirements for IoT systems. Over time, Archy becomes a knowledgeable assistant steeped in the context of your business.
From a strategic viewpoint, accelerating the requirements-to-backlog phase gives companies a huge competitive edge. It speeds up the delivery of customer value, because you’re spending less time in analysis paralysis and more time coding, testing, and iterating on actual features. In fast-moving markets, the winners are often those who can translate ideas into products quickest. AI-powered product management ensures that good ideas don’t languish in documents – they quickly become actionable plans. And because Archy integrates with tools like Jira, the output of this AI process flows directly into the execution pipeline . There’s no disconnect between planning and implementation; the AI-generated user stories can be immediately taken up by agile teams in their sprints.
To illustrate, imagine a cross-functional team at a large insurance company embarking on a new customer portal. Using Archy, the product leader gathers input from business stakeholders in a brainstorming meeting, then feeds the summarized needs into Archy. Within minutes, Archy produces a draft backlog: epics for user registration, policy lookup, claims submission, each broken down into user stories with criteria. The team reviews this draft the next day, tweaks a few stories, adds a couple of missed requirements – and by the end of the week, they have a complete, groomed backlog ready for development. In the past, this process might have taken 4–6 weeks of back-and-forth. Now, development can start in one week. Multiply that acceleration by many projects, and it translates to a drastically shortened time-to-value for the enterprise’s initiatives. The organization can deliver improvements to customers faster and respond more swiftly to new opportunities or regulatory changes.
Enterprise-Ready Flexibility and Governance
When comparing generative AI platforms, it’s crucial to assess their enterprise readiness – are they built to meet the complex needs of integration, security, and governance in a large organization? Many generative AI tools on the market today originated in the consumer or startup space; they may not check all the boxes an enterprise requires. Architech’s Archy platform, on the other hand, was designed from day one with enterprise concerns in mind. From deployment model to governance features, it aligns with what IT architects and CISO teams expect.
When comparing generative AI platforms, it’s crucial to assess their enterprise readiness – are they built to meet the complex needs of integration, security, and governance in a large organization? Many generative AI tools on the market today originated in the consumer or startup space; they may not check all the boxes an enterprise requires. Architech’s Archy platform, on the other hand, was designed from day one with enterprise concerns in mind. From deployment model to governance features, it aligns with what IT architects and CISO teams expect.
Security & Compliance
As discussed, Archy can be deployed as a self-hosted solution within your cloud environment, providing complete control over data access . All data processed by Archy stays within your network boundary, and you can enforce encryption, identity management (e.g. Azure AD integration), and any specific compliance controls you need. This is a stark contrast to many SaaS AI tools where you have limited visibility into their security measures. Archy also supports data isolation – teams or departments can operate in siloed workspaces if needed, preventing any inadvertent data mixing between projects . This is useful for maintaining Chinese walls or dealing with different data classification levels. Because it leverages your existing cloud’s security infrastructure, Archy effectively “inherits” compliance with standards your organization already meets. For example, if your Azure setup is HIPAA-compliant, Archy running in that same environment can be part of your compliance boundary. This alignment with enterprise compliance regimes means adopting Archy doesn’t introduce new headaches for risk management; it conforms to established policies and can be audited like any other internal system.
Integration & Extensibility
Enterprise IT environments are heterogeneous. Archy’s open architecture ensures it can interface with a variety of systems and data sources. In addition to Jira and Confluent, it can integrate with databases, message queues, and other APIs as required . It also provides an API of its own for custom extensions. This level of extensibility is important if you have a unique internal tool that you want Archy to pull data from or push recommendations to. Many other GenAI platforms offer only limited integration points (perhaps a plugin here or there), whereas Archy is positioning itself as a platform you can extend. This is closer to how big enterprise software like ERP systems or ITSM platforms operate – they provide rich integration capabilities so you can fit them into your environment. For AI to be truly transformative, it cannot live in a vacuum; Archy’s integration-minded design is a significant differentiator for enterprise use.
Governance & Control
With AI systems, governance includes monitoring usage, managing model versions, and controlling outcomes (preventing inappropriate content, etc.). Because Archy runs within your purview, you can log and monitor all AI interactions using your existing tools (e.g., Azure Monitor, Grafana dashboards) . This means you can trace how a requirement got turned into a user story or who accepted an AI-suggested change – invaluable for audit trails and understanding AI-driven decisions. Archy supports centralized monitoring and analytics so that administrators can oversee performance and usage patterns . If needed, you can establish guardrails – for instance, disallowing Archy from using certain data, or configuring it to require human approval before certain actions. Such fine-grained control is often not possible with off-the-shelf AI services. Moreover, owning the platform gives you control over the AI lifecycle: you decide when to upgrade models, apply patches, or retire capabilities. This avoids situations where a vendor might deprecate a feature you rely on or unilaterally change model behavior. In short, Archy’s model of “full control” means your team is in the driver’s seat for how AI operates within your business .
Finally, it’s worth noting that Archy is a new entrant in this space – it is currently in early adoption stages, with initial pilots rather than public case studies. This is not a drawback so much as a reflection of where the industry is as a whole. Generative AI for enterprises is a very recent development; in fact, studies have shown that over 60% of enterprise generative AI investments are still coming from innovation budgets, highlighting that we’re in the early stages of adoption . Early adopters of Archy are essentially partnering in exploring this frontier. The encouraging part is that Archy’s architecture and feature set were explicitly crafted to meet enterprise requirements, even before large-scale production deployments. It stands on solid, proven foundations (Azure cloud services, Atlassian ecosystem integration, etc.), which gives confidence that it can scale and comply with enterprise demands as usage grows. In other words, Archy has enterprise DNA – even as it continues to mature through real-world use, it was built with the right principles (flexibility, security, governance) from the ground up.
Embracing the AI Platform Advantage
As generative AI becomes a cornerstone of digital transformation, enterprises that own their AI platforms will be better positioned to lead. The strategic advantages are clear: you gain the agility to innovate without waiting on vendors, the security of keeping data in-house, the flexibility to choose and tailor models, and the seamless integration of AI into your existing operations. Instead of being bottlenecked by one-size-fits-all solutions or worrying about data exposure, you can focus on delivering value – like accelerating product development cycles and unlocking new insights – with confidence that your AI is working for you on your terms.
Architech’s Archy platform exemplifies this approach. It provides an open, extensible AI assistant that slots into your enterprise toolkit, enhancing it with generative AI superpowers while respecting the sovereignty of your data and processes. With Archy, product leaders can rapidly turn ideas into execution, and organizations can gradually scale AI adoption from pilot projects to enterprise-wide programs. All of this is achieved in a governed, secure manner befitting serious business applications.
While Archy and platforms like it are still in their nascent stages, the direction is set: enterprise AI will thrive on openness, integration, and ownership. The early adopters taking this path are essentially future-proofing their AI strategy. They will be able to incorporate the latest AI breakthroughs swiftly, because their foundation is adaptable. They will avoid the pain of vendor lock-in or compliance surprises, because they kept control from the start. And they will cultivate invaluable institutional AI knowledge, because their teams work directly with the models and data, not through a distant service.
In an era where every company is becoming an AI company, owning your AI platform is fast becoming not just a technical decision, but a strategic imperative. It’s about building AI capability as a core competency of your organization. Those who do so will have the freedom to innovate and the confidence to push AI to new frontiers, all while safeguarding the values and assets that make their business unique. In the long run, that is a defining competitive advantage. As you consider your enterprise’s AI journey, ask yourself: Are we content being users of someone else’s AI, or do we want to be owners of our AI destiny? The answer could determine who leads and who lags in the generative AI era. Embracing an open, owned AI platform like Archy might just be the key to unlocking sustained leadership and success in this next chapter of enterprise technology.